The Eyjafjallajökull Event:
What complex network theory can say
The eruption of the
Icelandic subglacial volcano Eyjafjallajökull in April 2010
threw a massive cloud of volcanic ash into the atmosphere,
and its subsequent dispersal blocked all air traffic in
many major European airports such as Heathrow, Charles de
Gaulle, and Frankfurt. In all more than 20 of the largest
European airports were completely shut down for a period of
several days.
Under normal conditions these airports serve some 1.5
million passengers in total every day, and passengers
travel to more than 1,000 international airports worldwide.
The complete shutdown of these airports thus represents a
significant impact on international traffic: thousands of
travelers are stranded at airports or unable to travel, and
the economic impact on the affected airlines alone is
around $200–250 million each day the airports remain
inoperative. The consequences of the ash cloud are also not
localized. Like many other technological or biological
systems, the global aviation grid is a large and complex
network in which even small changes may lead to unexpected
consequences for the network as a whole, and indeed
airports around the entire world are significantly affected
by the shutdown of the European centers.
Figure 1a: Shortest-path tree of Atlanta, USA
Move mouse into picture to see the impact of the
airport closures or download full-size pictures
before and
after closures.
Figure 1b: Shortest-path tree of Mumbai, India
Move mouse into picture to see the impact of the
airport closures or download full-size pictures
before and
after closures.
The conventional method for investigating the behavior of a
network subject to vertex removal is percolation theory.
This approach focuses on determining the number of nodes
that must be removed, through a targeted attack or random
failure, to split the network apart into small components.
However, in a highly-connected network such as the global
aviation network, even a removal algorithm that targets the
most important nodes must remove about 20% before the
network breaks down, equivalent to removing more than 80%
of the total traffic (see Fig. S2 at the right).
Although the eruption of Eyjafjallajökull has had a major
impact on air traffic, it has not brought the network close
to the percolation threshold (shutting down 27 major
European airports affected by the ash cloud on April 16,
2010 removes roughly 18% of the world-wide traffic).
Nonetheless, current events have crippled certain parts of
the aviation network, and by employing novel techniques
that take into account the varying strengths of connections
and local structure, we are investigating the impact on the
aviation system from a local perspective to gain a more
detailed picture of how the network structure changes.
Airport closings cause changes in effective
inter-regional distances
The core of our method is the
calculation of shortest paths in the network. When you use
Google Maps to get directions, it does not (necessarily)
give you the shortest route in terms of physical distance.
Instead, it tries to find a route that will take the least
amount of time; in this sense, two cities connected by a
highway can be “closer” together than two villages
connected by only a small road, even though both the cities
and the villages are equally distant in geographical terms.
Similarly, in the aviation network we are not interested in
the geographically shortest path. Instead, we say that two
airports are “close” when they have many passengers
traveling between them, and "distant" if they exchange few
passengers. Thus, this distance measures an effective
interaction between two airports (or the populations they
serve). One way to visualize this is to pick a reference
airport and lay out all remaining airports radially around
the central one. The distance from the central airport,
however, corresponds to the shortest-path distance and not
physical distance.
Figure 1a shows such a structured tree view of the
network from Atlanta International Airport. The lines
indicate the branches of the so-called shortest-path tree
that gives an impression of the structure of the whole
network as seen from the airport in the center, and the
colors of the airports indicate geographical divisions; all
airports in North America, for example, are dark-blue.
Note how the large red circle marks the approximate
distance of the world from Atlanta – only a few
large international hubs like Frankfurt (FRA),
London (LHR) or Hong Kong (HKG) lie inside the
circle while the majority of airports are outside. The only
exception, not too surprising, are the many North American
airports. Thus, the U.S. is “close” to Atlanta, while the
rest of the world is further, but equally far away.
Things change if we take out 27 major European airports
(move the mouse over Fig. 1a). The picture appears to
be less compact, indicating that the distance of many parts
of the world to Atlanta has increased. Indeed, all
West-European airports (purple) are now clearly outside the
circle. But also Africa (red, green, yellow), India (cyan)
and the Middle East (dark-purple) are being pushed away.
Furthermore, we can see structural changes: Normally, the
Chicago (ORD) branch contains almost all of Western
Europe, and in that the large London (LHR) branch
hosts Africa, India and the Middle East. Since London is
closed, Western Europe is now split into three parts behind
New York (JFK), Chicago, and Miami. Africa, India
and the Middle East are now largely in the Asian branches,
behind Honk Kong and Bangkok (BKK).
Similar observations can be made for many other airports.
To measure these changes quantitatively, we can compute the
relative increases in shortest-path lengths caused by the
closure of the 27 airports. The top panel of Figure 2
shows this for five selected airports, gathered by
geographic region. For example, the leftmost bar indicates
that the median of the relative increases of all the
shortest paths beginning at Atlanta and terminating in
Central Asia is about 35%. The bottom panel generalizes
this to compare entire regions against one another.
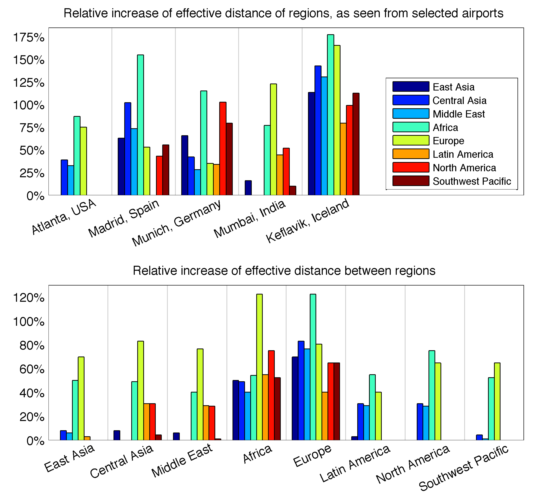
Figure 2: Relative increase of effective distances
in the network
Ot
her interesting case studies
are the German airport Munich (MUC) and
Iceland's international airport Keflavik (KEF).
Here, the world drifts away more homogeneously with
the closure of airports. This indicates that both
airports strongly rely on these now-closed hubs to
reach any part of the world. Contrary to Keflavik
though, Munich's distance to Europe increases less
than the rest of the world, meaning that while
dependent on hubs for global destinations, it is
well-connected locally within Europe.
Shortest-path-tree analysis reveals and quantifies
the effect of airport closures on global traffic
Figure 3: Shortest-path tree of Atlanta, USA,
geographically embedded
Move mouse into picture to see the impact of the
airport closures or download full-size pictures
be
fore and af
ter closures.
In addition to measuring the relative change in path
lengths, it's also possible to make a direct comparison of
the shortest-path tree of an airport before and after the
eruption, that is, to directly compare the structure of the
two trees of Fig. 1a or 1b. Every airport in the world
experiences some small effect: if you want to fly to
London, well, now you can't. Some airports, however, see
little change beyond the local shutdowns – you
can no longer fly to areas of Europe, but the shortest
paths to all other locations remain unchanged. These
airports receive a difference score of (nearly) 0. On
the other hand, airports that rely on a single strong
connection to a regional hub to serve large areas
experience massive changes in their shortest-path tree if
that hub closes, and the airports that experience the
strongest effect are assigned a difference score of 1.
The table below lists the 20 airports in the world that
serve the largest number of passengers daily in our data,
and orders them according to how much their shortest-path
tree is affected by the closing of airports in Europe. Many
airports are more severely affected than the ones in this
table; here we only look at high-traffic airports. As you
can see, many of the world's largest airports are
significantly affected by the events in Europe, despite
being geographically distant. In particular Singapore, Hong
Kong, and Beijing are all strongly affected, despite being
separated from Iceland by continents.
Code |
Name |
Country |
Difference Score |
MAD |
Madrid Barajas Apt |
Spain |
0.730 |
SIN |
Singapore Changi Apt |
Singapore |
0.423 |
JFK |
New York J F Kennedy International Apt |
USA |
0.231 |
HKG |
Hong Kong International Apt |
Hong Kong |
0.167 |
PEK |
Beijing Capital Apt |
China |
0.167 |
BKK |
Bangkok Suvarnabhumi International Apt |
Thailand |
0.128 |
DTW |
Detroit Wayne County |
USA |
0.115 |
ORD |
Chicago O'Hare International Apt |
USA |
0.115 |
PHL |
Philadelphia International Apt |
USA |
0.115 |
HND |
Tokyo Haneda Apt |
Japan |
0.103 |
EWR |
Newark Liberty International Apt |
USA |
0.090 |
IAH |
Houston George Bush Intercontinental
Apt |
USA |
0.077 |
DEN |
Denver Intl Apt |
USA |
0.038 |
DFW |
Dallas/Fort Worth Intl Apt |
USA |
0.038 |
LAS |
Las Vegas McCarran International Apt |
USA |
0.038 |
LAX |
Los Angeles International Apt |
USA |
0.038 |
PHX |
Phoenix Sky Harbor Intl Apt. |
USA |
0.038 |
SFO |
San Francisco International Apt |
USA |
0.038 |
ATL |
Atlanta Hartsfield-Jackson Intl Apt |
USA |
0.026 |
NRT |
Tokyo Narita Apt |
Japan |
0.026 |